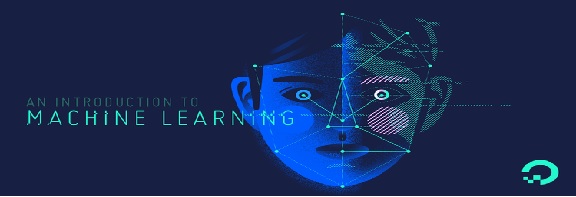
In 1997, The Asian Financial crisis was around the corner, but nobody could foresee the disaster. In 2000, the dotcom bubble was burst, it caused huge amount of loss. In 2003, SARS epidemic spread all around the world, it heavily affected the operation of stock and future markets and crashed many stocks markets. Stock market crash repeated itself over and over again two or several years right after previous one. So, it is normal to doubt that whether there are any signs or symptoms that can allow human to predict its coming. Hang Sang Index is a good indicator to predict the health status of Hong Kong stock market. Hang Sang Index has 50 constituents and all of them are considered as the most valuable companies in Hong Kong market. In these 50 constituents, there are 27 traditionally Hong Kong firms in utilities, finance, real estate and trading. When the stock market crashes, Hang Sang Index will follow and continuously fall. Hence, prediction of Hang Sang Index is still one of important task for investors to evaluate the health status of Hong Kong stock market. In this paper, a new and modern technologies, machine learning and deep learning, is introduced to investigate the movement of stocks. Since different stocks have different variables that are able to affect the variation of stock prices, this paper only focuses on around two or three field of stocks. For example, oil stocks are easily affected by oil prices, but oil prices are not the variable that affect the variation of stocks in real estate field or technology field. Exchange rates are easily waves the stock prices of export or import stocks and financial stocks, but it is not the same story for real estate stock in Hong Kong. In those selected field, around ten stocks will be chosen for investigation of stock prices. Some variables such as relevant news regarding the companies, public sentiments and past stock prices and any other related consideration will be analyzed through deep learning techniques. This paper is to use deep learning as a means to withdraw some relevant variables from investigating variables that fluctuates the stocks prices. The variation of stock prices is complex and the variables that able to affect the movement of stocks may have a little bit change over time. Hence, some correction techniques will be explored and introduced in this paper.